Rethinking Organizational Structures for GenAI-Driven Data Management
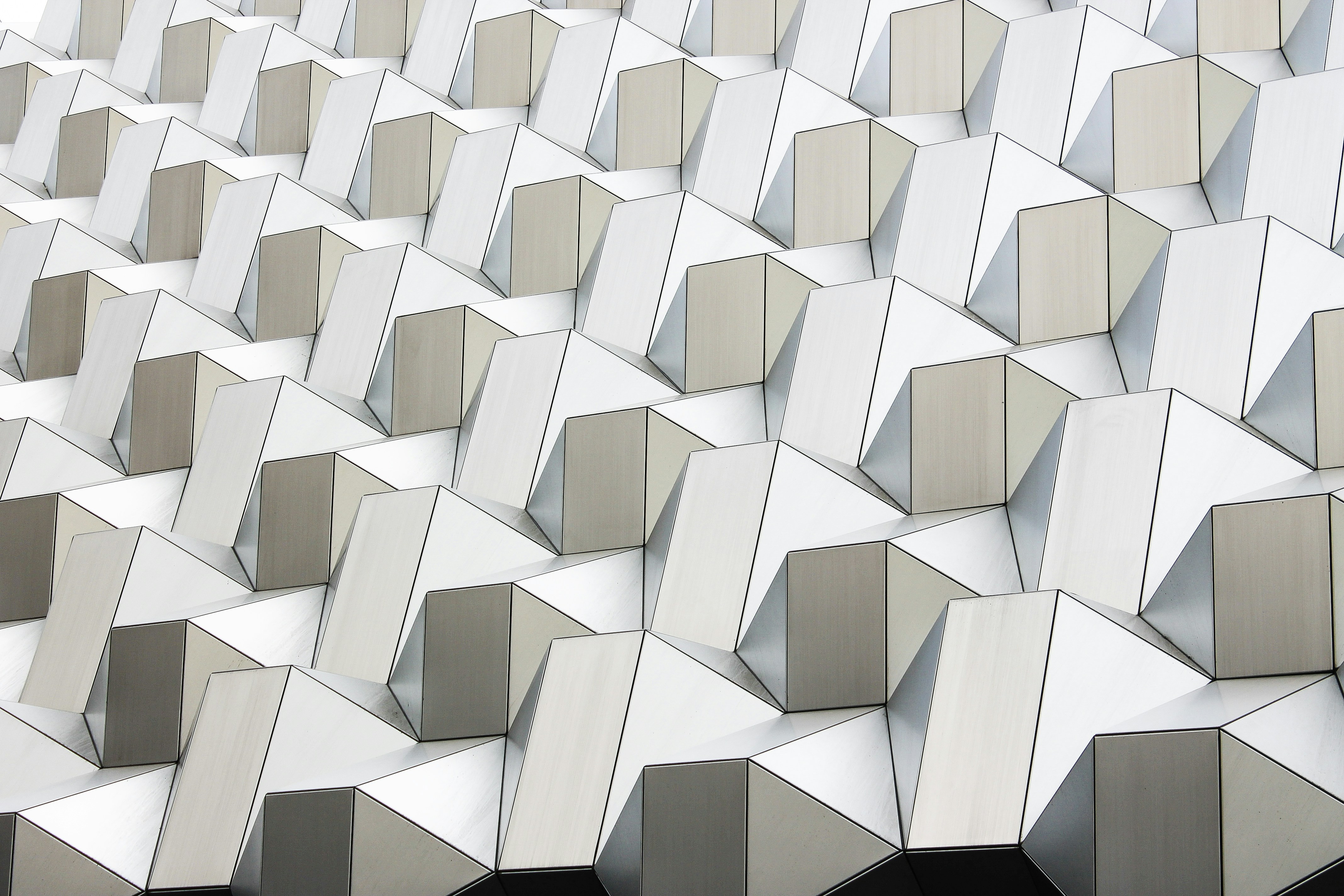
The Traditional Model: Data Silos and Hierarchies
"In the end, the success of AI in any organization will depend on how well it is integrated into the fabric of the business." – Satya Nadella, CEO of Microsoft
Traditionally, our approach to managing data has mirrored hierarchical organizational structures. We've created specialized teams, each focusing on a specific aspect of data management:
- Data engineers build and maintain our data infrastructure
- Analysts interpret data for various business needs
- IT teams ensure data security and system reliability
- Specialized teams (like actuaries in insurance or clinical researchers in pharma) develop complex models
This structure made sense in a world where data tasks were clearly defined and rarely overlapped. But in today's AI-driven landscape, these divisions are becoming more of a hindrance than a help.
For instance, at a major pharmacy chain, the inventory management team might be sitting on a goldmine of data that could revolutionize the marketing team's customer segmentation process. But because these teams operate in separate silos, with different goals and data access, this opportunity goes unnoticed.
The AI-Driven Shift: Flatter, More Integrated Teams
As AI takes over many of the routine data tasks, we're seeing a shift towards flatter, more integrated team structures. This transformation is manifesting in several key ways:
- Cross-Functional Data Teams: Instead of rigid departmental divisions, organizations are forming flexible, project-based teams that bring together diverse skill sets. For example, a large hospital looking to improve its patient outcomes might create a team that includes:
- A data engineer to handle complex patient data pipelines
- A machine learning expert to develop AI models for predicting readmission risks
- A clinical specialist who understands the nuances of patient care
- A UI/UX designer to ensure the solution is user-friendly for healthcare providers
- Emergence of Citizen Data Scientists: With AI making data analysis more accessible, we're seeing the rise of "citizen data scientists" – professionals who can perform simple data science tasks, even if it's not their primary role. In a retail setting, this might mean store managers using AI-powered tools to perform their own data analysis on inventory turnover and customer foot traffic, rather than always relying on a separate analytics team.
- AI as a Team Member: Increasingly, AI isn't just a tool used by human teams – it's becoming an integral part of the team itself. Consider a pharmaceutical company's drug discovery department. AI algorithms might continuously analyze molecular structures and biological interactions, flagging potential drug candidates for human researchers to review.
- Emphasis on Soft Skills: As AI takes over more technical tasks, there's a growing emphasis on uniquely human skills like creativity, critical thinking, and communication. In an insurance claims department, for instance, while AI might handle the initial assessment of straightforward claims, human adjusters are now freed up to focus on complex cases that require empathy, negotiation skills, and nuanced judgment.
Real-World Success Stories
These shifts aren't just theoretical – real-world success stories are already emerging, particularly in the banking sector:
- Document Automation: Several commercial banks are using GenAI tools to automate the preparation, aggregation, and validation of documents for trade finance, know your customer (KYC) efforts, and compliance reporting.
- Code Development: Banks are allowing developers to use GenAI to help write code that supports various banking activities.
- Investment Assistance: JPMorgan Chase has filed a patent application for a GenAI service that can help investors select equities.
- Bond Market Intelligence: Tools like Broadridge's BondGPT offer investors and traders answers to bond-related questions, insights on real-time liquidity, and more.
- Wealth Management: Morgan Stanley has built an AI assistant using GPT-4 to help its wealth managers quickly find and synthesize answers from a massive internal knowledge base, summarize client meetings, and generate follow-up emails.
While these success stories are encouraging, the transition to AI-integrated teams is not without its challenges.
Challenges in Restructuring
Organizations face several hurdles as they adapt to this new paradigm:
- Resistance to Change: Organizational inertia can impede transformation. McKinsey reports that 70% of transformations fail due to resistance. For example, a risk analyst at a commercial bank who's spent years honing their SQL skills might be hesitant to embrace a new AI-driven approach to credit risk assessment.
- Skills Gap: AI's rise demands new skill sets. A Salesforce survey indicates that 62% of employees feel they need additional skills to work with AI effectively. Healthcare providers, for instance, are finding they need to invest heavily in training medical staff to work alongside AI systems, blending traditional medical knowledge with data science concepts.
- Data Governance: As data democratization increases, ensuring data quality and security becomes crucial. Retailers need to balance the benefits of data accessibility with stringent customer privacy requirements.
- Cultural Shift: Transitioning to a collaborative model requires cultural transformation. Getting traditional pharmacists to collaborate closely with data scientists on AI-driven drug interaction models can be a significant cultural challenge.
The Path Forward
Despite these challenges, organizations can adopt several strategies to navigate this transition successfully:
- Start Small: Begin with pilot projects promoting cross-functional collaboration. A regional grocery chain might start by creating a small team to develop an AI-driven inventory management system for their produce department.
- Invest in Training: Develop data literacy and AI skills across all levels, partnering with educational institutions if necessary. A large hospital network might create a program training nurses in AI concepts related to patient care.
- Rethink Incentives: Align rewards with collaborative achievements rather than individual performance. A pharmaceutical company might reward researchers for effective AI tool usage in drug discovery processes.
- Lead by Example: Leaders should embrace AI-driven insights in decision-making, setting a precedent for their teams. For example, learn how the CEO of a large pharmaceutical company has pioneered AI use with Clarista to accelerate strategic decisions-making.
- Maintain Flexibility: Foster an adaptable organization that evolves with technological changes. An insurance company might adopt an agile approach to its organizational structure, regularly reassessing and adjusting team compositions based on emerging AI capabilities and business needs.
As we move forward in this AI-driven era, the most successful organizations will be those that can harness the power of both human and artificial intelligence, creating structures that allow for seamless collaboration between the two. In our next and final post of this series, we'll explore how to cultivate a culture of curiosity and continuous learning across industries – the true key to thriving in the age of AI-driven data management.
Photo by Silvio Kundt on Unsplash